Optimisation is everywhere
-
How should we manage inventory to avoid stockouts and write-downs?
-
How should we schedule staff to save costs and meet demand?
-
How should we set prices to maximise margin?
As a business manager, if you are trying to answer similar questions, you are not alone. Organisations everywhere regularly ask operating questions like these to improve their business performance. Across a wide range of industries, businesses are becoming smarter and more cost-efficient in the way they run their business.
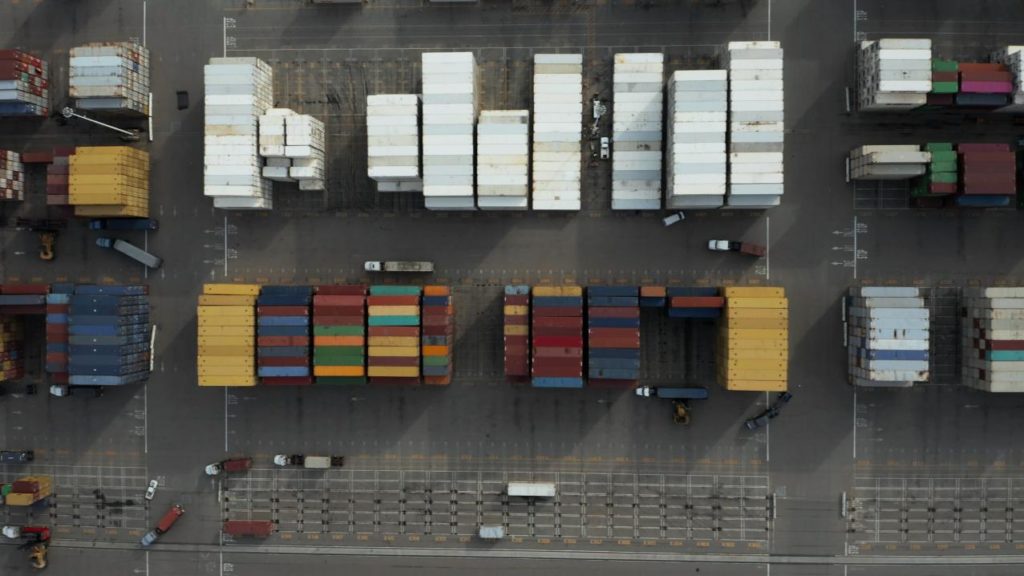
Optimisation is the process of finding and implementing new ways of making your business more efficient, more productive and (consequently) more profitable. In a competitive, dynamic market driven economy, the ability to make complex decisions in an accurate and timely manner can have a huge impact upon a business’s bottom line.
Using organisational data and advanced analytical techniques, the process of optimisation aims to identify alternative ways of doing things – resulting in higher profits through either lower costs, increased sales, or a combination of both.
What is Optimisation?
Optimisation is about efficiency and productivity – taking a system (existing or planned) and finding an ideal way to run aspects of the operations. When many possible approaches to a business problem exist, with no straightforward method of choosing the best one, optimisation is a powerful tool that improves the viability of a business by providing marginal gains.
Optimisation does not replace domain expertise, but rather complements it – a vital ingredient in the process is a dialog between the data scientists creating the optimisation and the business stakeholders. Implementing optimisation can allow businesses to improve performance by minimising costs, efficiently allocating resources, increasing sales volumes, and identifying critical points or bottlenecks.
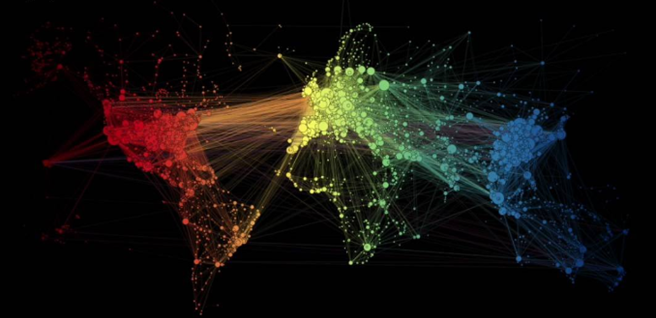
Case Study: Biofuel Supply Chain
Forecast recently ran an optimisation for a bioenergy company engaged in establishing a biorefinery in rural Australia. The business takes offcuts from crops grown in the surrounding area and to recycle into useful materials – primarily natural gas.
The supply chain optimisation focused on the acquisition and logistics for the delivery of offcuts to the biorefinery. Every year, over 300,000 tonnes of raw material need to be stored, transported, and pre-processed before being converted into biofuel. The company required an optimisation model to provide timely information about the most efficient and cost-effective way to organise this process, while as the same time having the flexibility to run various scenarios based on weather conditions and crop yields.
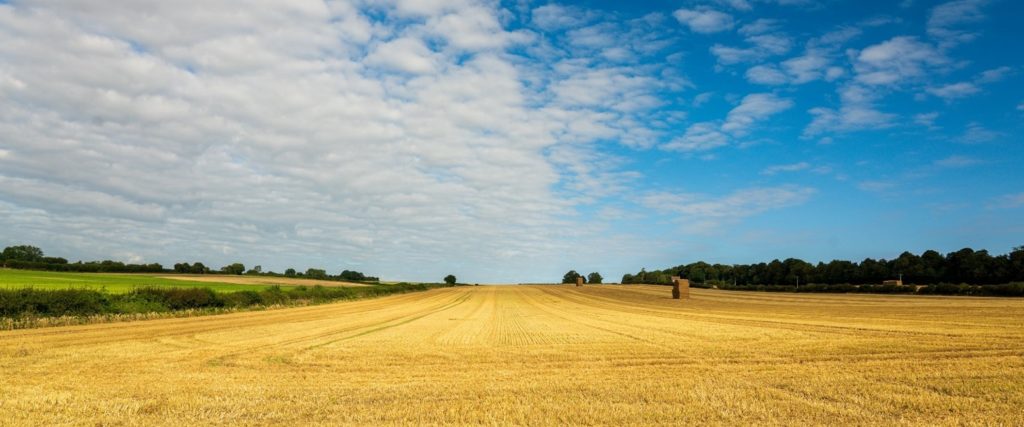
Forecast delivered an optimisation model that provided the company with a blueprint for their ongoing business operations. This model was centred around organising crop acquisition from local farmers, storage, transport, pre-processing, and delivery to the biorefinery – while at the same time minimising the total costs associated with the process. The model’s transport and storage schedule guides the weekly operations management, and the cost estimates are used to enhance their financial modelling.
It was important that the model be both reusable and robust to changing conditions. As such, we designed the optimisation process to be transferrable to various geographic locations, as well as be adaptive to changes in agricultural land use patterns. The company can update inputs as real-world conditions change, and for similar future bioenergy projects within their portfolio.
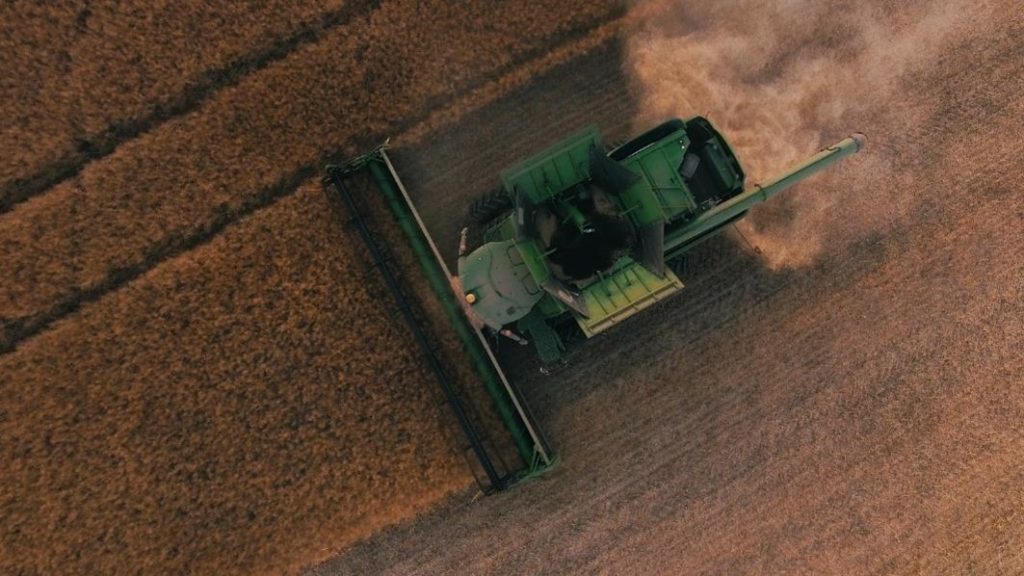
The success of this project highlights the benefit of a bespoke approach – the ongoing dialog between Forecast and the business stakeholders allowed an understanding of the project to develop. Whilst off-the-shelf products may be available, these typically involve a trade-off between standardisation (does not reflect the real world), or customisation (expensive and prone to error).
Approach to Optimisation
The design of an optimisation model begins by scoping the problem with the data scientists and key business stakeholders, gaining a qualitative understanding of the real-world system that will be optimised. This process usually involves several meetings or workshops to focus on the relevant operational and commercial information.
Once the problem has been defined, we translate these real-world systems into the language of mathematics. Most problems can be described in a set of linear equations, the most important of which is an objective function – the problem that needs to be minimised or maximised. In practice, the objective function usually revolves around either minimising the total cost of a process, or maximising the total profitability.
Most of the work in setting up an optimisation problem is in defining constraints. Constraints are limits on the real-world system, which can come from physical limits, regulations, or commercial obligations. Transport offers a useful example here – there are physical limits on how much can be carried by a truck, but there are also legal maximums on size and weight.
After the mathematical definitions have been confirmed, they can be converted into software code to run the optimisation calculations.
Successful optimisation models are based upon engagement with key stakeholders. They are the experts in their specific domain, and will have an understanding of the problem, costs, and limits that can’t be gained within the timeframe of a project.
Critically, experience tells us that optimisation models should be set up with a flexible approach – all parameters are potentially adjustable to suit the business needs. This allows the model to be updated to respond to new information, and for the model to be used to test the potential impact of future shocks or different scenarios.
-
Prioritising Speed versus Complexity
Most optimisation problems have an inherent level of complexity that can lead to a model taking a long time to converge on a solution. Although speed can be improved by writing efficient code, and the application of cloud computing (see below), there is often a trade-off between speed and complexity. The best way to approach this is dependent on use case. In general, an optimisation that is run once can afford to be slower to increase accuracy; but an optimisation that needs to be run multiple times (for instance to test multiple scenarios) necessitates more of a focus on speed.
-
Application of Cloud Computing
Cloud solutions, such as Amazon Web Services, Google Cloud, and Microsoft Azure can be used to provide more computing power, and therefore reach a solution faster. These are paid services, but the performance gains are large. Ensuring that the model is scalable when writing the code makes the transition to one of these platforms near seamless.
-
Complementary Data Sources
Any optimisation needs to be related back to real world outcomes. Often a step in this process is linking the results of an optimisation to existing data sources. Depending on the use case, this may be internal data collected during day-to-day operations, or external data sources to describe the conditions the business is operating in.
In transport and supply chain projects, the key complementary data source is usually geospatial data – data that relates to maps and other location descriptions. It is useful both as an input (e.g. distance to transport) and output (e.g. storage locations). There are several open-source government data files that provide rich geospatial data. After some data transformation, the geospatial data can be linked into the optimisation, calculating the distance between locations, and therefore the most efficient transport schedule.
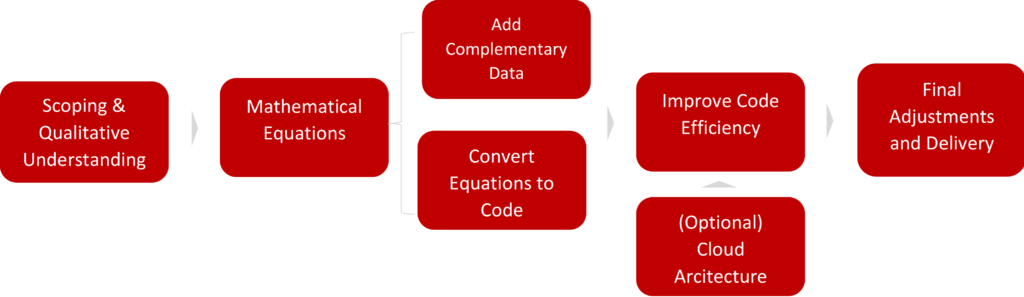
The concept of Optimisation is applied to different types of businesses and various aspects of their operations.
-
Staff Rostering
Rostering is a challenge faced by many organisations that schedule work around shifts, such as retail stores, call centres, and hospitality venues. Salaries are often one of the highest operating costs, hence underutilised staff are a major challenge. However, missed sales or poor service creates a burden on revenue and long-term business performance. The use of optimisation allows these organisations to reduce labour costs while still providing a target customer service level.
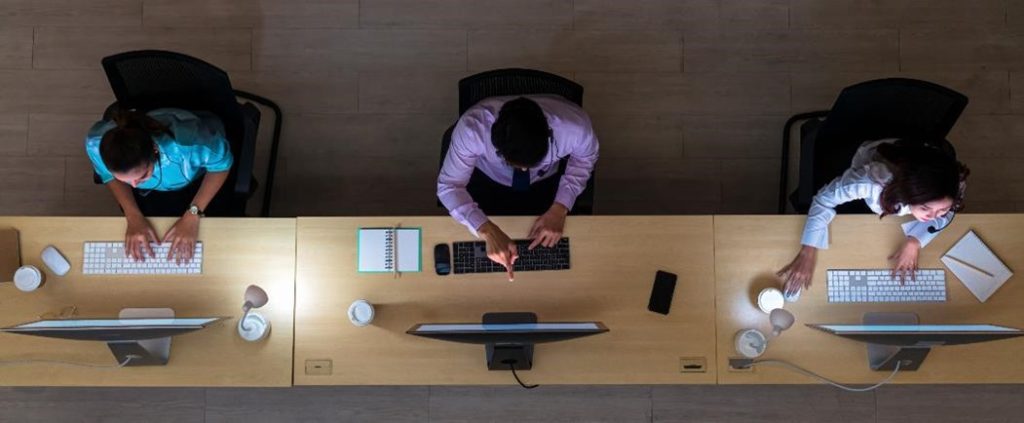
-
Transport Scheduling
Transport is a classic example of an optimisation problem. For many products, particularly bulky products with long supply chains, the cost of delivery from manufacture to end customer can contribute a large percentage of the total cost of sales. Given a set of destinations, costs, and restrictions on travel, optimisation can define the most efficient way to schedule movement of products across various forms of transport.
-
Inventory Management
Managing any physical inventory is a balancing act, with downsides associated with both over-ordering (obsolete stock and high storage costs), and under-ordering (missed sales opportunities and frustrated customers). Optimisation assists businesses in defining order quantities and timing to provide confidence that stock will be available when required, while minimising the disposal or discounting of surplus stock.
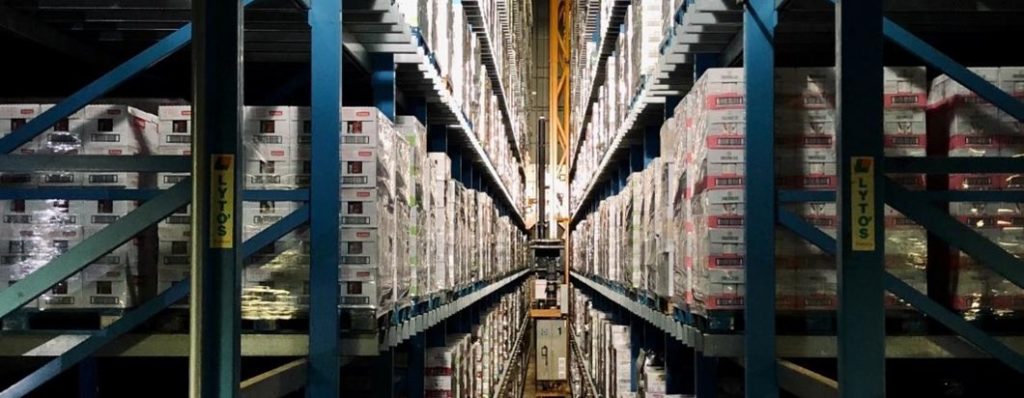
-
Price Optimisation
Setting the optimal price and calendar of promotions for your products or services can be very time consuming and lead to sub-optimal outcomes. An essential element of tackling price optimisation involves forecasting demand for products and services with confidence. Once the demand is understood, an optimisation exercise can help determine the optimal price, given constraints on availability and costs.
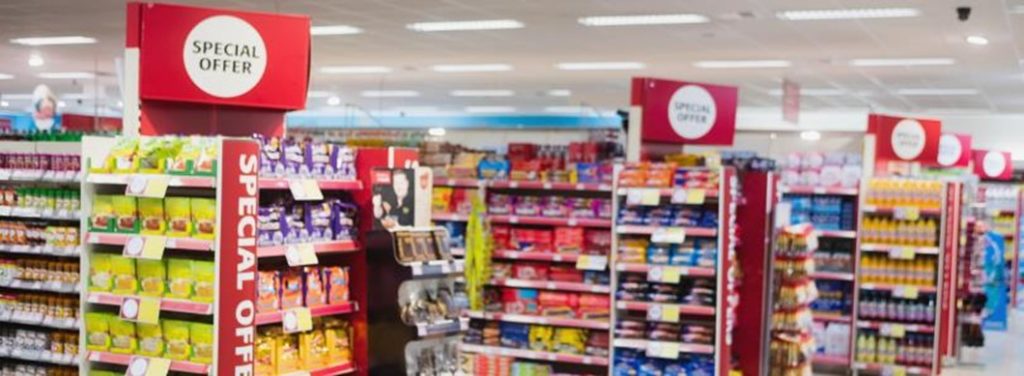