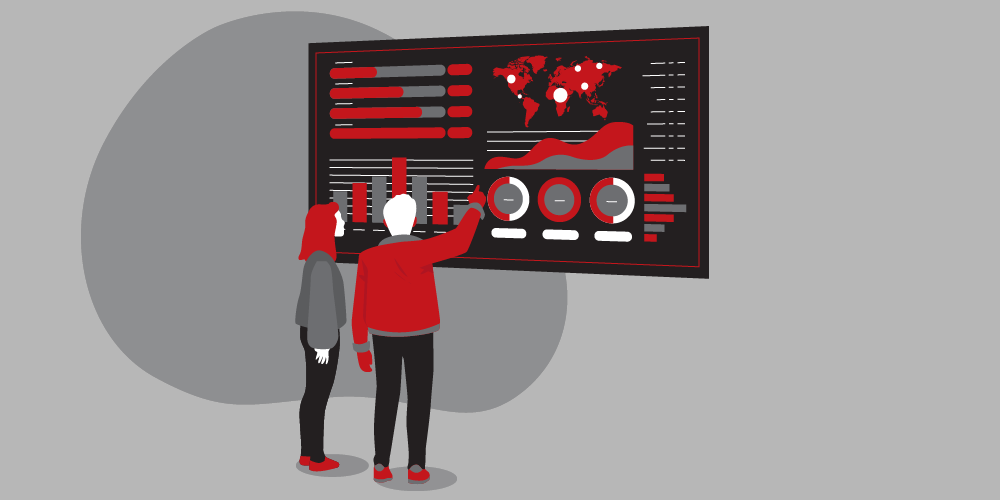
The term ‘scientist’ tends to conjure up images of someone in a laboratory making hypotheses and testing them. Data scientists are not too different – similarly, they make their best guesses at how data behaves in the real world.
The Harvard Business Review famously proclaimed that the role of a data scientist was the “sexiest job of the 21st Century,” as data has become one of the world’s most valuable commodities and, as LinkedIn’s CEO, Jeff Weiner, once said “Data really powers everything that we do.” A data scientist understands how to harness data to learn more about businesses and their customers.
So how does this differ from an exact science? Data science will likely never be an exact science as it is only as strong as its weakest link. The quality of the data used is critical, and the involvement of humans at various points in the process introduces subjectivity and challenges related to interpreting and translating commercial objectives and, critically, communicating results effectively. A robust, end-to-end approach can help mitigate some of these challenges.
“Data really powers everything that we do.”
Jeff Weiner, LinkedIn CEO
Data science still includes experimental design, data gathering, conducting the experiment and, most importantly, communicating the results. If the hypotheses being tested are successful, they become models that explain and predict. However, it could still be argued that there is no “science” to this. As in economics, a given approach to answering a question about something as complex as behaviour can be highly subjective or multi-faceted. There is no single way that customer data can be used in marketing campaigns or recommendation systems. Additionally, predicting the likelihood of customer churn because of a price increase or attempting to isolate individuals who may commit credit card fraud is not simply solved with a single model.
Ultimately, the models and methods used are only as good as the creativity of the data scientist in defining their inputs and the available data, and data quality can vary significantly between organisations. This is why it is critical that internal company resources, or external experts, recognise the importance of embracing an end-to-end approach, including having the right data and engineering infrastructure in place, as well as being able to apply a commercial lens to delivering actionable insights.
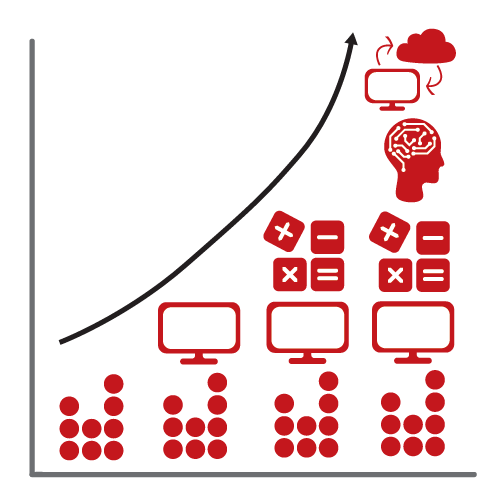
That said, technical skills still matter greatly. In what is a varied role, data scientists are very much in demand in industry as they are valuable across many divisions within businesses, e.g. finance, marketing, technology, and operational sectors. It is difficult to constrain the technical skills included in the role, but programming, statistics, software engineering and business intelligence are all common components. These technical skills can be broken down even further, such as the programming language used (e.g. R, Python, Java, Go), and even the different packages within them (e.g. sklearn, tidyverse, pandas in Python). There is also the key skill of being able to translate from the non-technical to the technical, and vice versa, as business objectives need to be converted into a (scientific) model and then delivered as an interpretable result to learn or improve on a part of the business.
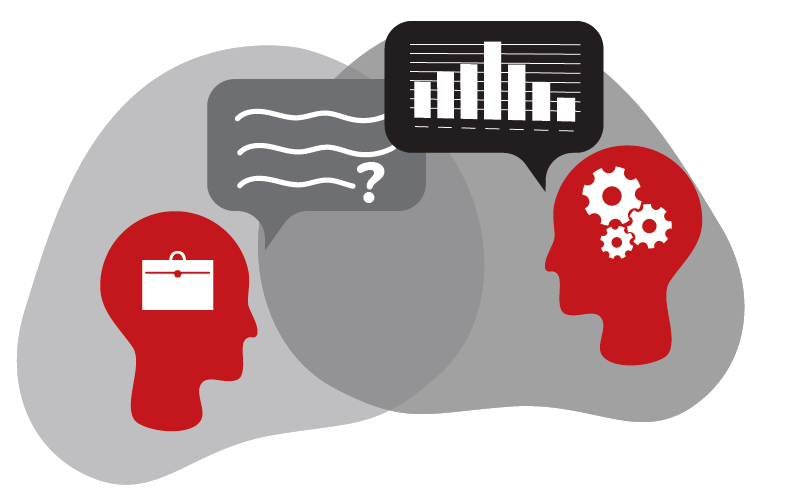
If data science were an exact science, there would not be the need for such a broad range of skills. Some of the individual activities within the role are scientific, such as the experimental methods and models in algorithms, some are purely engineering (e.g. for the design and development in the software engineering), some are primarily mathematical, and some hinge on the data scientist’s communication skills and commercial acumen. But most of the time, the work is a combination of all of the above. There is no “one size fits all” approach to the work of a data scientist.
There is not a “one size fits all” approach to the work of a data scientist.
This implies that using the newest and most complex deep learning model would not necessarily guarantee one becoming the best data scientist. Rather, as Dr Jacqueline Nolis, a data science leader in the Seattle area who helps Fortune 500 companies, discusses in the DataCamp Podcast, the ability to make good PowerPoint slides is arguably more important, as communicating results is critical to the role. This connects with the skill of business intelligence – there is no use in creating a complex model for a business if they cannot use or understand it.
Moreover, as Ronald H. Coase, a renowned British Economist famously once said, “if you torture the data long enough, it will confess.” This emphasises the importance of having clean and transparent data when training so that the fitted model can be appropriately validated in a way that minimises any inherent bias in the model. This is critical to ensure that any business decisions can be taken with confidence. Data scientists require that this business intelligence not only gives an accurate prediction or forecast, but also provides an insight into the business problem itself. In learning from the past, businesses can better prepare for the future.
“Science is a way of thinking much more than it is a body of knowledge”
Carl Sagan
If we were to use Carl Sagan’s quote of “Science is a way of thinking much more than it is a body of knowledge,” then the way the data scientist thinks is very much scientific. In reality, however, data science is not an exact science as humans are not exact creatures. But we as humans can hone the skills needed to ensure that data science is as impactful as it can be.