Embracing computer-assisted decision making will give early adopters a competitive advantage through faster processing time, fewer errors and enhanced customer experience. Insurers can achieve better claims management by using the intelligent technologies in some of the following ways.
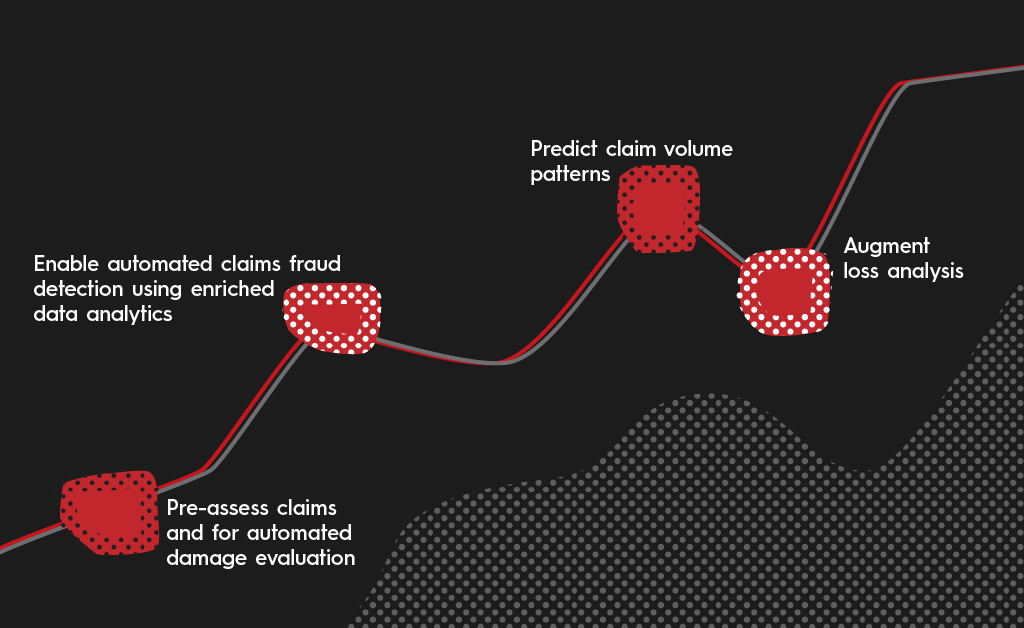
The Insurance Technology Vision 2019 by Accenture reports how more than 75%of insurance leaders acknowledge their employees and consumers are more mature when it comes to ‘digital’, as insurers themselves are slow-moving due to their size. This leads to a workforce embracing new technology ahead of their employers, paving the way for InsurTech. This has largely been externally driven, compared to some of the so-called ‘in-house disruptors’ we tend to see in banking and telecommunications.
Telematics and Usage-based Pricing
Traditionally the insurance industry has been a pioneer of using mathematics in risk estimation and pricing; their ability to embrace new techniques and alternative data sources early on might give them a competitive edge in years to come.
Telematics is one of those alternative data sources that remains an interesting topic for motor carriers. Even though it has been around for many years, it has evolved considerably since its introduction, certainly not living up to the hype it once created, and now entering a phase of realism. This age of realism is where genuine use cases start to appear. Usage-based pricing is seemingly closely related to telematics and describes the concept of allowing the persistent monitoring of risk exposure during the coverage period enabling insurers to price each risk at the most adequate rate. The use of telematics has been largely restricted to an upfront discount, behavioural monitoring during the cover period and adjustment upon renewal.
Research by the Casualty Actuarial Society Insurance On-Demand Working Party shows how 89 percent of consumers said they would rather pay a single, stable price per year compared with paying per usage without a certainty of total price. Usage-based motor insurance will be favoured by consumers penalised by traditional insurance products, that is, consumers with low claim rates that would otherwise have to pay for more coverage than they need.
Self-selection has had its impact on telematics-based products globally. Two customers seemingly identical based on their characteristics traditionally considered for pricing, the one who accepts the telematics product offering has lower probability of generating a loss. The most robust study comes from Italy, the country with the largest proportion of new policies sharing telematics data with the insurer. One study shows how this risk self-selection effect accounts for 20 percent of the claim frequency, which is tremendous. Not the actual telematics data themselves, but the choice whether to accept telematics can now be used as an indicator in more traditional pricing models, prior to coverage; i.e. penalised for not choosing telematics.
Other than risk self-selection, there are plenty other use cases that are exploited by insurers who are re-inventing their claims processing through telematics. It’s helping companies use data to support the claims processing by using collision trajectory to determine fault. Interesting fraudulent claims about imaginary crashes or people who stage claims can also be easily rejected through locational data. The Association of British Insurers disclosed how motor insurance fraud is most prevalent, with £629 million of detected dishonest claims.
In addition to the use of telematics data, computer vision is also transforming claims processing and associated fraud analytics.
One example of this type of Artificial Intelligence includes Ageas and Tractable who deployed an AI-assisted claims handling following a pilot in the fall of 2016 that visually inspects car damage based on consumer provided images in order to provide estimates and generate approvals for repairs. Earlier this year Tractable shared some of their insights and challenges around the real-world deployment of claims processing systems assisted by their technology. Automation of workflows leads to a reduction in the resources spent on managing claims, increasing process efficiency and boosting the customer experience.
Prevention is better than the cure
As new data sources are increasingly more accessible through the Internet of Things (IoT), insurers will be able to move beyond the traditional reactive model of paying claims after a loss and adopt a proactive, preventive business model of avoiding losses in the first place by encouraging behavioural changes or continuous risk monitoring.
Commercial property insurers can use the data generated by smart buildings to help their customers reduce the risk of fire or water damage, and the data generated by telematics allows customers to get feedback on their driving behaviour. TheFloow and MyDrive are two examples of InsurTech players that go after this market in their own way. Similarly, US life insurance John Hancock announced that all newly written policies in its Vitality programme would require policy holders to wear an Apple Watch, rewarding users upfront. The collected data could prove vital in their future underwriting business.