Protecting Your Modelling Strategy Against ‘Model Drift’ Beyond Covid-19
Who would have thought six months ago that tourism companies would suffer a sudden drop in holiday bookings to the most popular destinations in Europe (after the UK introduced quarantine)? Or that plastic surgeons would face a dramatic increase in demand for soft-tissue fillers due to face masks being part of the ‘new normal’?
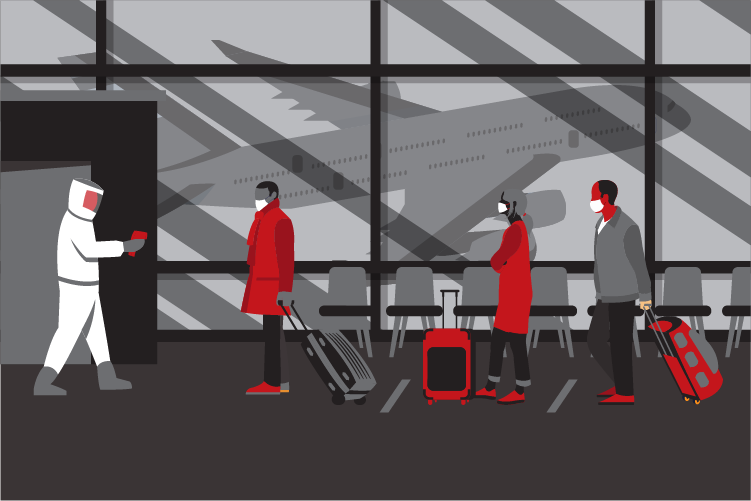
The effect of the Covid-19 pandemic on the way we live and operate is unavoidable. Data science, like everything else, has also been affected. The previous examples show how a disruptive event (Covid-19) can cause a significant loss in the ability to predict. This concept is commonly known as ‘model drift’.
So, what exactly is ‘model drift’? It is concisely described as when
“the relationship between the target variable and the independent variables changes with time”
Although this phenomenon is not new, Covid-19 has put it back on the map for many in the analytics world. It has, across the board, highlighted the need for re-thinking the way businesses should future-proof their data science projects.
This blog post will present the two main scenarios in which you might find model drift and how to deal with them. Finally, it will provide some recommendations for making your data science projects robust to model drift.
Case 1: Model assumptions and data have changed
This is the most disruptive scenario for your models. It will possibly have affected all aspects of the modelling procedure. Some examples are:
- The input data sources have changed drastically,
- The assumptions behind what features help predict your target variable no longer hold,
- The meaning of your target variable has changed,
- The appearance of hidden features is affecting the relationship between your predictors and your target variable.
This case scenario involves a complete revision of all the steps that make up your pipeline.
An illustrative example of this can be the prediction of traffic during March-July 2020 in my hometown, Barcelona.
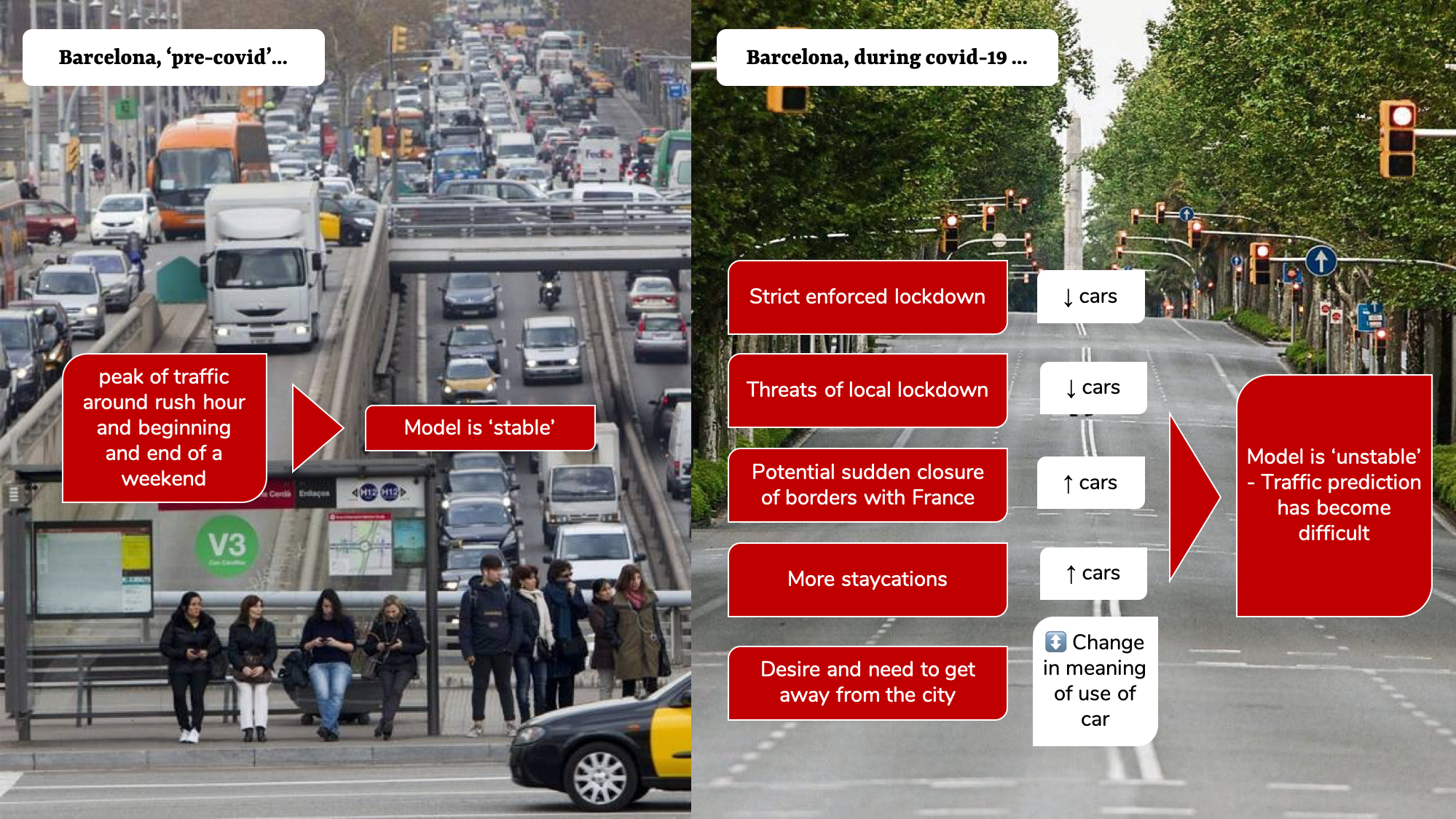
Case 2: Model assumptions remains the same, but data has changed
In this second scenario, we don’t expect the underlying relationship between predictors and target to change. However, the input data that is being fed into the model has changed over time. This can be due to:
- The distribution of the input parameters has shifted
- Business updates have changed how input parameters are defined.
In this case, it will require a re-training and possibly re-mapping of the inputs in the model to account for the drift. A couple of examples of changes in data inputs could be:
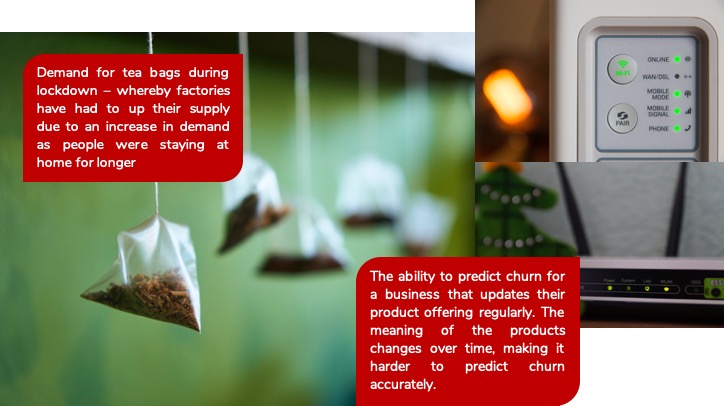
In some cases, the changes in the data can be due to modifications upstream in the data pipeline. Changing the pre-processing step is likely to have an impact on your input features.
Making your processes more robust to model drift
As we have seen in the previous case scenarios, different types of model drift will require different approaches. Some tips to ensure you are protecting your models from drift:
- Good documentation of the process – including assumptions, data sources, statistical properties of the data and model predictions.
- Fluid communication with the teams supplying the data or deciding on business assumptions will also help catch issues before they become a problem.
- Regularly monitor your model predictions, your input data and your data labelling over time. By defining a set of metrics and tracking their trends over time, it is possible to pick up differences that would be hard to spot from just a snapshot. Platforms such as Domino Model Monitor or Databricks are good examples of, increasingly popular, monitoring solutions in the market.
(Image is from DOMINO website)
- Deploy your processes in such a way that a regular re-fit of models can be done with certain frequency. Decisions in this aspect will include scheduling re-trainings or thinking about weighting the inputs of your model based on recency of data. Cloud platform providers such as AWS (through SageMaker) or Azure (through Data Studio) are now offering automatic model re-training based on alert systems.
- Choose the right data science platform – with automation in mind! Older data science approaches generally require more checks and manual work but will have a tighter grip on making changes. However, as companies productionise their models, automation allows for less human effort on data quality-checks and more time delivering value to the business.
Everything in life, including models, deteriorates over time. The phenomenon known as ‘model drift’ occurs in different scenarios and will require distinct strategies to mitigate it. However, good documentation and an integrated implementation of the process will go a long way in detecting and dealing with model drift.